ECE Dept. Head Leading NSF Grant to Secure and Accelerate Machine Learning
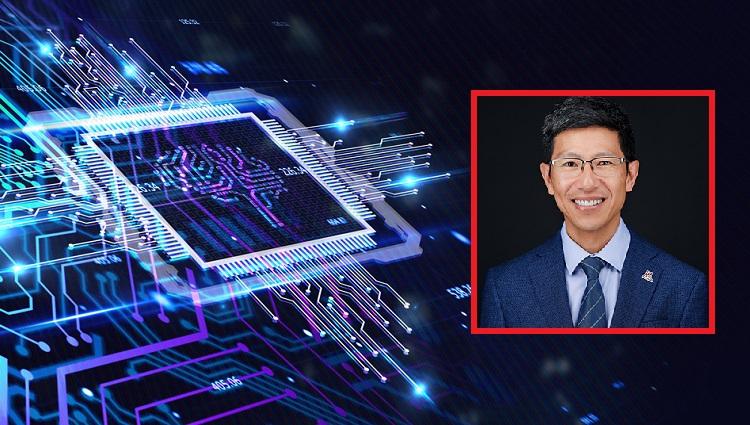
While machine learning is bringing exceptional advancements to computing, its powerful nature makes it an attractive target for cybercriminals. Additionally, its interdisciplinary potential can lead to a steep learning curve. Electrical and Computer Engineering Department Head and professor Hongyi "Michael" Wu recently earned a National Science Foundation grant to develop a system that will serve as a toolbox to accelerate research in machine learning security and privacy.
With this $1.3 million grant, the University of Arizona will work with the University at Buffalo to develop hardware and software for DEEPSECURE: A Development and Experimental Environment for Privacy-preserving and Secure Machine Learning Research. Like a toolbox, DEEPSECURE contains a number of useful devices while also remaining an enclosed space. As principal investigator, Wu will lead the UA’s $721,000 portion of the grant.
"Security is a never-ending battle. We have learned this through human history,” Wu said. “DEEPSECURE will facilitate a variety of research projects that focus on machine learning security and privacy, ranging from privacy-preserved training and inference to adversarial attacks and defense.”
Machine learning is a crucial tool in everything from engineering to finance to homeland security, but Wu says most machine learning research focuses on performance, not security. He argues that security in machine learning is falling behind – an issue that if not soon addressed will become a fundamental hurdle in its development.
To help fix this, DEEPSECURE involves both software and hardware. Wu's project will build a hardware infrastructure that runs DEEPSECURE. Researchers can then use it as a secure cloud service. But it will also be offered as a software toolbox for users who want to download it to their local servers. This novel approach will produce customizable, yet ready-to-use building blocks that flatten the learning curve for researchers coming from both the machine learning and security communities, Wu said.
For the hardware platform, Wu foresees a scalable computing environment based on the latest Dell, AMD and Nvidia GPU technologies to establish the DEEPSECURE hardware infrastructure across the campuses of UA and UB. The software toolbox will be integrated with the machine learning framework PyTorch for usability for both beginners and advanced researchers.
Community, Outreach and Collaboration
Beyond the creation of the DEEPSECURE system, Wu's project also seeks to develop a community bridging machine learning and security. And the NSF grant will be used to promote DEEPSECURE across the nation to foster a self-sustainable machine learning security and privacy research community. Beyond this, he also seeks to train a diverse workforce to safeguard the future of intelligent systems.
This effort will be facilitated by a committee working with the project team to develop plans for inspiring and improving the participation of groups who are underrepresented in science. This outreach will include open forums, training workshops and visits to historically black colleges and universities.
"In addition to offering training opportunities to college students, researchers, and practitioners, the project team will reach out to high school students, especially students with low socioeconomic status … to introduce cybersecurity and AI career path and educational resources to K-12 school counselors, teachers, students, and parents,” Wu said.
Outreach opportunities include the existing annual NSF-supported GenCyber summer camps for K-12 students and the Cyber Saturday series.
The NSF grant and project are being shared with the University at Buffalo partly to optimally integrate experts from the fields of machine learning and security. Two university sites will allow the team to build a networked DEEPSECURE platform to investigate security issues in a distributed machine learning system.
The DEEPSECURE platform is intended to support research opportunities and foster a long-term ecosystem for machine learning development. However, a number of ongoing machine learning projects can benefit from this, such as security in health care systems, hands-on lab learning against cyber attacks, and privacy in neural architecture search.
"The success of this endeavor will lead to breakthroughs to secure machine learning systems, accordingly accelerating their development and widening their adoption in various science, engineering, medical, finance and homeland security applications,” Wu said. “We welcome all researchers and users who care about machine learning security and privacy to be part of the project.”