Tracking Cancer Growth with AI
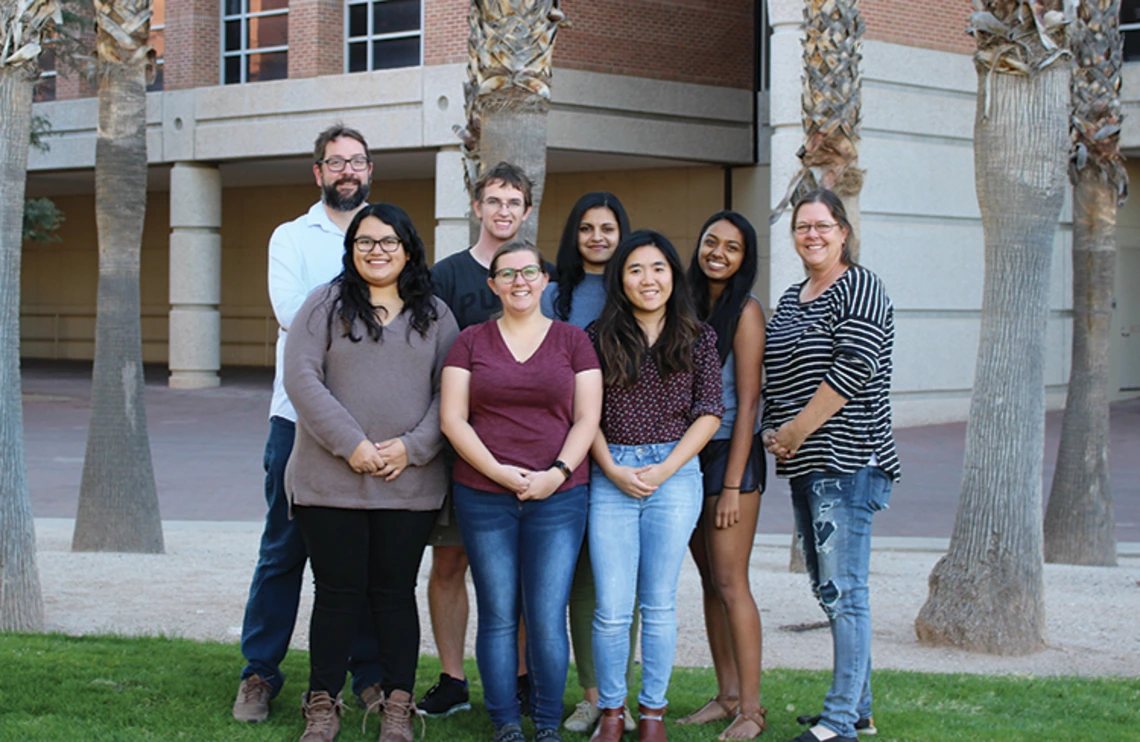
Artificial intelligence algorithms and sophisticated sensors come together as researchers seek to identify cancer earlier and stop it from spreading.
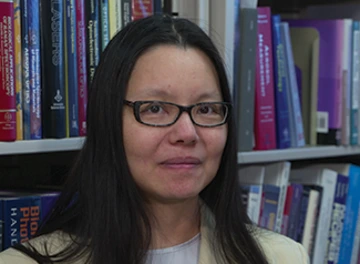
Janet Meiling Roveda, who holds dual appointments in electrical and computer engineering and biomedical engineering, is using artificial intelligence to speed up the process for tracking the way breast cancer and colon cancer cells morph and spread. Ultimately the research is expected to improve cancer treatment.
“Right now, it’s all done by humans,” Roveda said. “People are using microscopes to take photos, and from those images, they identify and manually mark the cancer cells: Where are they located? When did they start to split? And once they split, how many turn into new cells or die? And if they split, who is whose ancestor? We realized the problem is right there: The humans are a bottleneck.”
This painstaking manual process of identifying and marking the cells on hundreds of individual slides takes the researchers an average of eight hours, often conducted over two or three days. Once the slides are treated, researchers take images until it is possible to identify movement. And once they’re done, they repeat the process. Not only is the process on each slide slow, but also the slides can only document dozens of cells at a time.
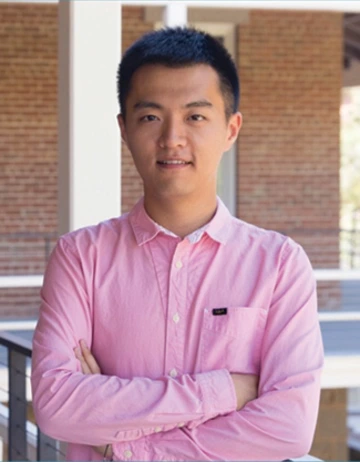
Roveda and Andrew Paek, an assistant professor of molecular and cellular biology, are using a Convolutional Neural Network, or CNN, a type of algorithm that can learn to recognize objects based on their shape. To teach CNN what to look for on the slides, Paek’s team manually tagged thousands of cells, and the algorithm learned to copy the process. The CNN identifies and marks cancerous cells in just 10 minutes, as opposed to eight hours. The artificial intelligence integrates with powerful advanced microscopes that can process dozens of cell slides at a time. Plus, the AI can view the cells in wavelengths undetectable to the human eye, providing even more information faster.
“Instead of using visible light, we’re using more channels – for example, fluorescence,” Roveda said. “Normally when you use fluorescent images, you have to translate them back into something the human eye can see. Now, we don’t need to do that.
Through the processing using AI, we can identify the cancer cells long before they have any visible morphology changes.”
The team has filed invention disclosures for some of the algorithms and plans to use them at the UArizona Cancer Center. Also contributing to the project was Siteng Chen, who earned his PhD in ECE in 2020 and now works as a data scientist at Esatto Healthcare.
Roveda is also the director of the Center to Stream Healthcare In Place, an academic and industrial partnership that aims to develop personalized in-home technologies for managing chronic diseases. Some of the center’s partners, including Baylor College of Medicine and the California Institute of Technology, also are interested in using the AI-cancer tracking technology in clinical practice.
“This is very reliant on collaboration,” said Roveda. “If we look forward, a lot of this is very likely to be used in the clinic where they measure the reactions to cancer treatments.”