When

Thursday, November 14, 2024 – 3:30 p.m.
Jason Pacheco
Assistant Professor of Computer Science
University of Arizona
"Fast and Flexible Information Estimation for Decision Making and Control"
ECE 530 | Zoom link
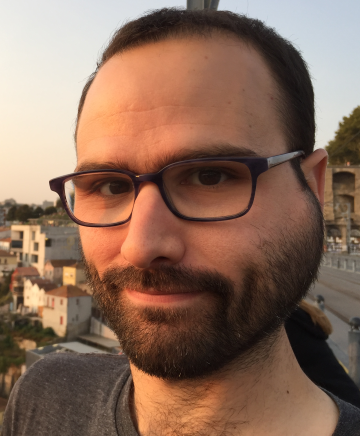
Abstract: In this talk I will discuss methods for estimating mutual information (MI) for sequential decision making and optimal control. MI is a commonly used measure of expected uncertainty reduction in an unknown quantity of interest. But despite its apparent utility MI lacks a closed form for most statistical models of practical interest and so must be estimated. I will discuss efficient methods for estimating MI based on variational inference techniques and flexible methods that incorporate normalizing flows estimation. I will investigate the use of these methods in the setting of Bayesian optimal experimental design (BOED) where the quantity of interest is fixed but unknown. Finally, I will consider an extension where an unknown quantity varies with time as a response to control inputs, as in the setting of active information acquisition (AIA).
Bio: Jason Pacheco is an assistant professor in the Department of Computer Science at the University of Arizona. Before joining UA Jason completed his PhD in computer science at Brown University and held a postdoc at MIT CSAIL. Jason's research interests in statistical machine learning aims to build intelligent systems for automated reasoning in stochastic environments. To achieve this requires the development of algorithms that yield efficient inferences, while remaining flexible enough to adapt to complex domains. Jason's research interest span a variety of application domains including computer vision, signal processing, computational biology, and computational neuroscience.